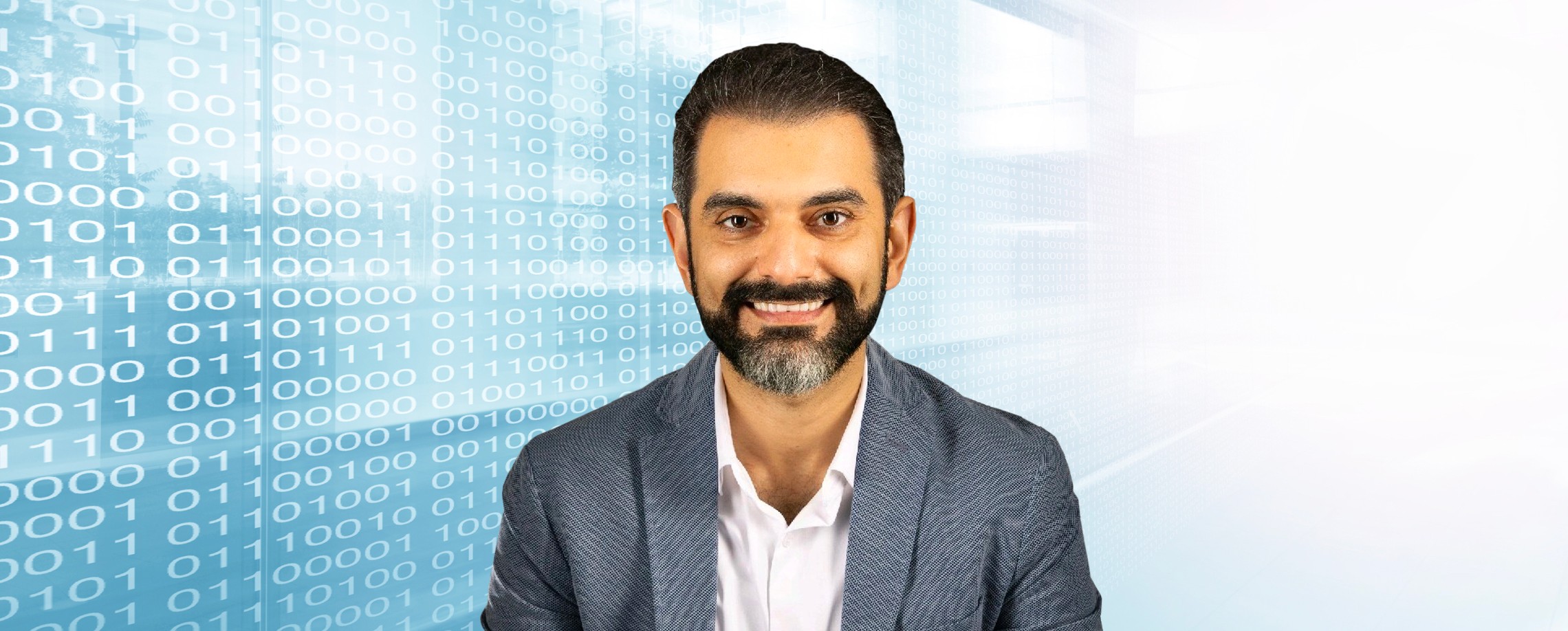
- Author: Tariq Munir | Digital Transformation Advisor
- Posted: May 8, 2025
3 Steps for CFOs to Adopt AI in 2025
We are all excited about AI. Conferences, articles, blogs, podcasts are filled with content around the capabilities of AI and its impact on the future of work—ironically often generated by AI. Terms like predictive analytics, generative AI, and lately, AI agents are being thrown around freely—and this is where the real problem lies.
The hype has rendered AI as a mere technological advancement as opposed to a leadership opportunity. We can easily come up with what problems AI can solve in general, but the real challenge lies in how to use this technology to address our specific business issues. In fact, I often get asked about how to even start with AI?
My response to such questions is simple—you DO NOT start with AI.
You start with the problem you are trying to solve. AI, or any other technology for that matter, is only one as good as the problem it can solve and is just one of the enablers to do so.
Having said that, AI is no-doubt one of the most powerful general-purpose technologies of today, and if used correctly, we can leverage it to fundamentally transform finance and business operations.
To do that, we need to create a balance between a longer-term digital strategy and short-term execution. Despite this article being a bit AI-centric—to help you kick-start your journey—none of the AI solutions should be falling outside your overall digital vision and strategy.
Now let’s explore how by using this three steps approach, you can start utilizing AI to solve some of the most pressing business problems. If done correctly, you should be able to go from identification to deployment within 3-6 months.
Step 1: AI Design Sprints
The fastest way to brainstorm, identify, and prioritize automation opportunities is through conducting an AI design sprint. Effectively, a cross functional team of domain experts (finance, commercial, operations), AI experts (internal or external), and internal IT and Data teams come together to brainstorm areas that are the biggest pain point for business.
An internal or external design sprint facilitator (who can also be the AI expert above) can help keep the discussion on-track and facilitate the right conversation. These sprints can last from anywhere between a couple of hours to a couple of days, depending upon the complexity and size of the business and its issues. The output is a high-level view of key pain points, the business value unlocked by solving them, data and infrastructure needs, and prioritization.
Now I strongly recommend having a digital council or an innovation board comprising of functional or executive heads representing business, IT, finance, legal etc. This board/council reviews these recommendations to ensure they are aligned with overall business direction, can be funded, and are within the ethical and responsibility guidelines.
The last point is especially important since it is easy to overlook the ethical implications in the favour of speed and agility. For example, do we have the right to use the customer data to train the algorithm.
Step 2: Proof-of-concept (POC)
Once you have aligned the top 2-3 priorities, the next phase is to build proof-of-concepts. This is where your internal IT team can help you shortlist a few vendors that are well suited for such development. Start with some well-established business processes for which relatively mature solutions are available in the market.
For example, instead of going right after agentic AI to automate accounts payable, start with traditional machine learning solutions to verify invoices and payments. Similarly, rather than focusing on your business-specific complexity, start with general finance problems like financial planning workflows, transaction processing, process optimization etc. These industry-agnostic problems have tried and tested solutions available in the market and generally have a fast speed to deployment.
Now a POC does not mean handing over the problem to an AI developer and follow up on the implementation. Finance teams need to work closely with the vendors throughout the AI development lifecycle. We have already covered the problem framing and business value part of this development lifecycle in Step 1. A crucial phase of AI model development is its training and testing. We effectively select a small sample of data from the entire population and split it in training and testing set. The training set, as the name suggest, is used for training the model and then testing set is used to validate the output of the AI model.
If we have not been careful in selecting training and test data, and it is not representative of the original population, this could mean an AI model can perform very well in a test environment but when exposed to real-world unseen data in production, it gives inaccurate results. This can also result in a model which is biased in its output as the model might learn only specific patterns as opposed to general
If you have correctly done the problem framing, data identification, and worked closely with AI developers in training and testing the model you should be able to bring the proof of concept to life within 3-6 months.
Step 3: Iterate and improve
Now this is the most important step, or sometimes I call a mindset change. AI is not a set-and-forget kind of traditional software implementation. The initial AI model you will deploy is likely to be the least accurate version.
However, it is nothing to worry about—at least for now. As the model is put to use, it will get exposed to new data and hence will learn patterns from the real-world data. This means we get some invaluable insights about the accuracy of the model. Listen to the feedback from stakeholders and front-end users of the model and keep iterating.
In AI development, more usage means more data, and more data means better model. It is a virtuous cycle of AI development. However, as finance leaders we must embrace agile mindset and instead of aiming for perfection, balance speed and accuracy to launch the minimum viable product and then improve that product in successive iterations.
In my experience, some good candidates for such 3–6-month AI proof of concept deployment can be AI-driven process optimization, building small process digital twins, account payable workflows, account receivable invoice matching, and reconciliations. However, it will depend on your specific business needs and priorities. These quick wins help you solicit support from the board and executive team to put more funding behind broader digital transformation. Moreover, these will help you build confidence in the output of AI and your teams will start feeling more comfortable working with it.
Remember, the intent is not to bring AI for the sake of it. It is a balancing act between longer-term strategy and short-term execution, while solving specific business problems. This does not mean we lose sight of the bigger picture.
Any digital solution, AI or no AI, must always remain anchored in an identified business need. With the right orchestration and framing of business value, there is no reason finance function cannot unlock true potential of AI.
About the Author – Tariq Munir
Tariq is a Digital Transformation advisor and consults businesses on unlocking potential of AI, Data, and Digital. He is also a keynote speaker, trainer, and monthly columnist at CFO Magazine A/NZ.
He can be reached at hello@tariqmunir.me or www.tariqmunir.me