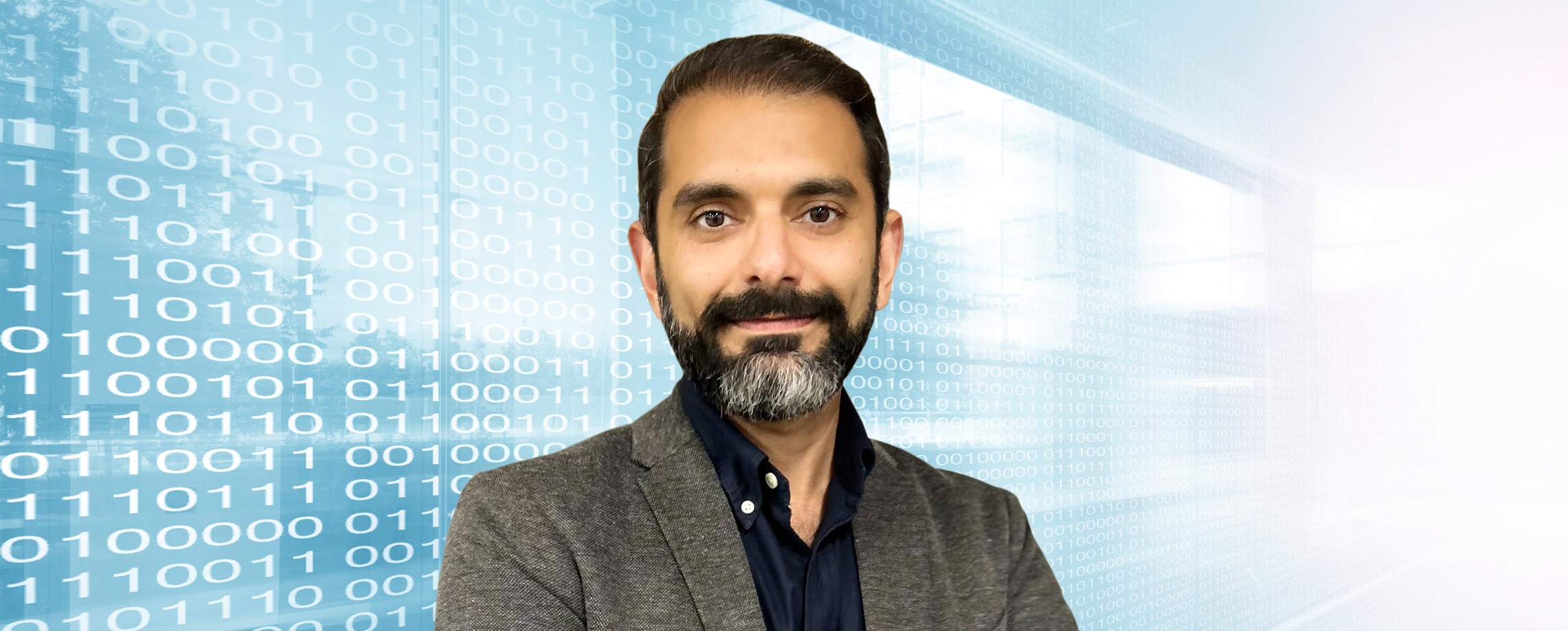
- Author: Tariq Munir
- Posted: January 28, 2025
2025 – The Year of Agentic AI…or maybe not?
Part 1: Understanding the Hype & 5 Real-life Examples
By now, I am sure you would have seen or heard enough about the next frontier in AI evolution — Agentic AI. It is the closest we are getting to autonomous AI capable of breaking down complex tasks into smaller problems and solving them almost independently — or with very few humans in the loop.
I briefly touched upon this in my last article for CFO Magazine, however given the hype, more needs to be written or rather explained about it.
This sudden hype is fuelled by a couple of factors. Firstly, Large Language Models (LLMs) are becoming increasingly sophisticated in reasoning and calculations, allowing them to handle complex tasks with fewer human touchpoints. Secondly, these models are no longer restricted to text inputs — they can work remarkably well with images, audio, and videos. These advancements are making it possible for multiple AI systems (or agents) to work together at once and solve complex, multi-step business problems.
Think of it that way. Generative AI is great at solving one specific task based on data it is trained on. For example, you can ask it to “generate an analysis of last month’s financial results” and it will do a relatively great job in doing so. It will analyse, create tables, and graphs, and even recommend a certain course of action. However, if you ask it something like “analyse results, generate next quarter’s forecast, and explain gap with the annual plan”, the output will be highly restricted to the data the Gen AI model has been trained on and most likely incorrect as it ignores any input from other functions like sales, marketing etc. Gen AI is great at acting as a co-pilot to humans.
However, as we move from co-pilot to full action-oriented AI, this same task by an agentic AI system will be done in a significantly independent and interconnected manner — much like humans. One agent will download actual results from ERP, and the other in the meantime gather inputs from the sales team on demand forecast, the production team on supply plans, and the marketing team on innovation launches. Another agent would collate all this information into a financial planning tool — consolidating it into a P&L. It would then pass it on to the Gen AI engine to create the new summaries and analysis based on this new input from various functions. Humans are in the loop during these performance review workflows only at critical points or validations. This autonomous orchestration of different agents is what makes this technology immensely powerful.
A Contrarian View
There is a bit of a conundrum here. Agentic AI is the next frontier and is becoming a reality due to the sheer potential of generative AI. However, businesses are still struggling to see any significant, game-changing impact of generative AI. This can be proved by the fact that — as I shared in some of my previous articles — 80% of AI projects are still falling short of their objectives. This raises several questions; is agentic AI something that is killing generative AI as we know it today, and how practical it is for organizations to bring agentic AI into their workflows considering we haven’t fully unlocked how to do that with the current generative AI models, or worst of all — is it just another hype?
This article is a two-part series that will address all these questions and discuss — from the CFO’s perspective — what needs to be done in 2025 to ensure AI (agentic or otherwise) is solving some of the most complex and pressing business and finance issues.
Let’s evaluate the first part of this problem. Where do we stand on agentic AI actual use cases front and how far is it in creating a tangible business impact?
Separating hype from reality
To understand the hype, let’s go back to basics for a moment. At the end of the day, agentic AI is not a standalone technology. It is based on all the other AI techniques working in collaboration with each other. One agent might be good at predictive analytics, another one at computer vision, and another one at generating and summarizing reports. Something we noticed in our earlier performance workflow example. What truly differentiates is the added agency to all these algorithms enabling an autonomous operation of these agents.
While still in its infancy, we can find numerous use cases and examples where agentic AI is already creating massive efficiencies for businesses. While some can argue if few of these are truly autonomous or not, nonetheless are good early examples to look at:
Alaska Airlines is building a natural language search that will provide a ‘travel agent’ -like experience for travellers to plan and manage their vacations and travel itineraries.
Blitzy.ai is a software development startup that employs agentic AI to build software codes. They recently built enterprise software with 30,000 lines of code, that would have taken six months. They did it in just six days with only one senior engineer in the loop.
Best Buy is developing a generative AI-powered virtual assistant that can enable troubleshooting any product issues, schedule deliveries of orders, and manage subscriptions — among other tasks.
Wiley, the global publisher, implemented AI agents to handle service call spikes. The solution outperformed their previous chatbot, increasing self-service efficiency by 40% and achieving 213% ROI. It is now developing a pilot “prompt builder” for faster, reusable prompting support for employees to complete tasks more efficiently.
Puma, the international sports brand, leverages agentic AI to create customised product photos for its website, enabling local market relevance. The technology has boosted PUMA India’s click-through rates by 10% — taking product personalisation to the next level.
These are just a few examples of how AI agents are being used currently. However, remember that Agentic AI is enabled by the convergence of numerous AI techniques like natural language processing, computer vision, predictive analytics, generative AI etc. It is the orchestration of these different AIs that makes agentic AI so powerful.
This also means that agentic AI would not be a fit for every business problem. Problems that are less complex and straightforward would still benefit more from traditional AI approaches. The ROI might not justify using AI agents to solve every multi-step problem and having a human in the loop might be a more feasible option.
What is next?
When we talk about potential, the above examples do not even scratch the surface. Virtually any workflow — even the most complex and dynamic ones — can be automated using agentic AI. In finance think of a multi-step month-end process, monthly rolling forecasts, stage-gate innovation process, financial reporting, and so on.
However, before we get too excited, there is a need to do a reality check. The underlying processes, workflows, and operating models in finance need to be redesigned before we can embrace agentic AI. Now, we can also not wait to fix everything first and then leverage technology. A complex interplay and balance between short-term objectives and longer-term strategy is needed.
In next month’s article, I will share what key steps CFOs need to take in 2025 to embed this agentic AI revolution into finance and register some early wins. Stay tuned!
About the Author – Tariq Munir
Tariq Munir is a thought leader on AI, Data, and its intersection with business. He is also a keynote speaker, trainer, and monthly columnist at CFO Magazine A/NZ.
He can be reached at hello@tariqmunir.me or www.tariqmunir.me