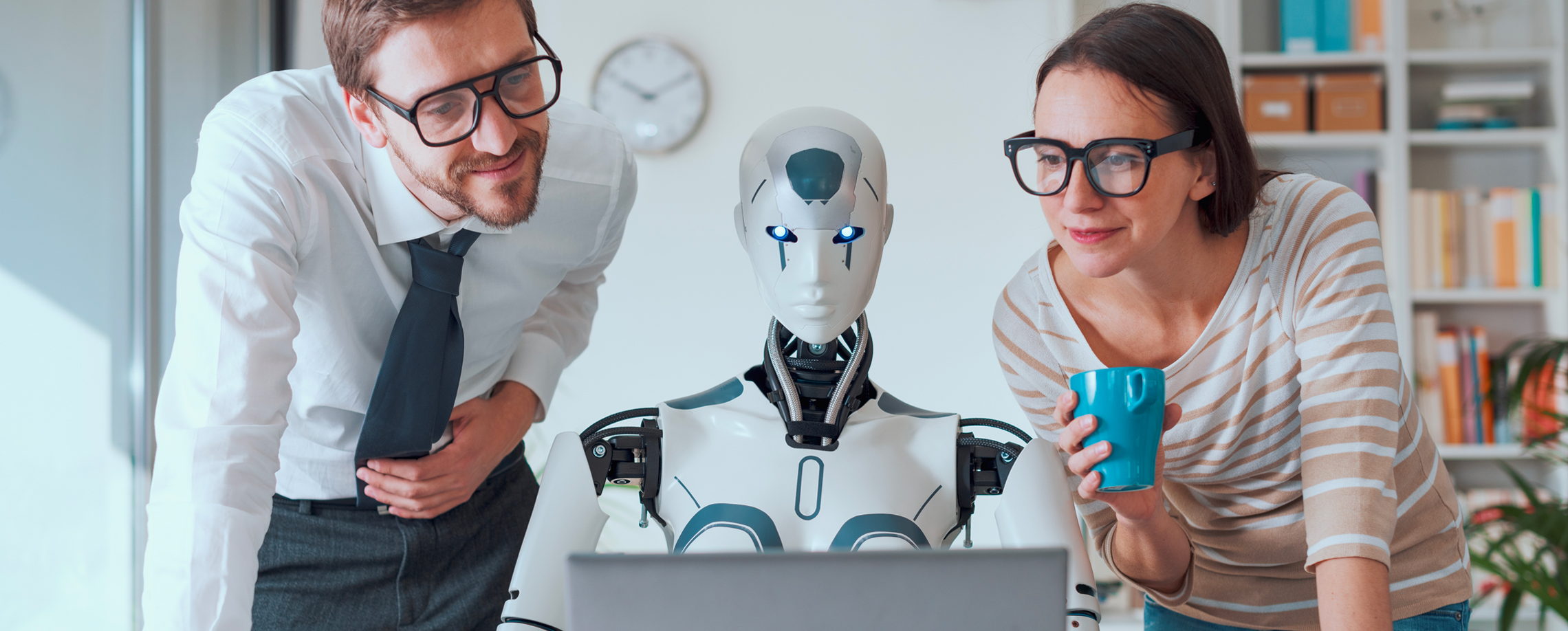
- Author: Mark Pesce | Author & Futurist
- Posted: July 18, 2023
The CFO’s New Trusted Assistant > Autonomous Agents
What could a ‘perfect assistant’ do for you? You’d be able to assign it any task, any responsibility, any role, and know that the assistant could perform admirably. A perfect assistant would understand the way you work – the way your organisation works – and work with it, improving your capacity to get things done. A perfect assistant would help shoulder the burden, making even the most difficult days in the office far easier.
In our idle moments we imagine that we only need to clone ourselves to create that perfect assistant. But a clone has a mind of its own – and might well have other ideas. The perfect assistant never disagrees, never argues over a point of pride or principle; they just get on with the job.
Then again, if the perfect assistant truly can do everything you request – does that make them a rival for your job? Would a perfect assistant be a bigger threat than opportunity? Maybe you don’t want a perfect assistant after all?
Too late: they’re now well on their way, under cover of another name, ‘autonomous agents’. Although this may sound like a brand-new thing, the history of autonomous agents stretches back more than thirty-five years, to a demo developed by Apple Computer. During Apple’s ‘lost’ years – between firing Steve Jobs (1985) and hiring him back again (1997) – a succession of CEOs tried to demonstrate their own flair for ‘inventing the future’. First cab off the rank, former PepsiCo President John Sculley, who described a hypothetical autonomous agent – dubbed the ‘Knowledge Navigator’ – in his business memoir Odyssey: Pepsi to Apple.
An accompanying video – written by Apple, but with a generous helping of Hollywood special effects – shows the Knowledge Navigator in use, helping a busy university professor handle his correspondence – while simultaneously assisting him to prepare for a lecture being delivered later that same day. The Knowledge Navigator chats away in plain English, listens and responds appropriately to plain English, and does pretty much everything asked of it – even going so far as to leave an ‘I’ve called’ note on the professor’s behalf when reaching out to a colleague who can not be contacted.
Watching the video you get a sense that this was really why Amazon, Google and Apple lavished so much money on Alexa, Google Assistant and Siri. Each sought to recreate the magic of the Knowledge Navigator. Despite billions of dollars and decades of research, none of those voice interfaces can do very much more than play a song or run a timer. The chasm between potential (as explored in Apple’s video) and reality haunted those technology giants. They all wanted the Knowledge Navigator – but none of them knew how to make it real.
Then along came ChatGPT. When powered by GPT-4 – the latest and by far the most capable of all of the ‘large language models’ – ChatGPT brings a nearly human-like sensibility and responsiveness to human interactions. ChatGPT delivers the richly human-like ‘agent’ the Knowledge Navigator first revealed to the world – and which Big Tech had never been able to provide.
After GPT-4 landed, it didn’t take long for an enterprising programmer to work out how to pop it into a framework that gave an autonomous agent most of the capacities of the Knowledge Navigator. It’s called Auto-GPT, and when you install it – it’s a bit of open source software that you can freely download and run on just about any PC or Mac – and fire it up, and poses you a question:
I want Auto-GPT to:
Whatever you type in response – however practical or fanciful – that’s what AutoGPT will try to do for you.
How does it perform this bit of magic? One of the things we’ve learned – after a century of both operations research and management theory – is how to break any activity into actionable parts. Auto-GPT will first translate whatever you’ve typed as a response into a goal. This it does by asking ChatGPT to do the heavy lifting. Auto-GPT itself is more of a conductor, it doesn’t try to interpret English-language requests, instead forwarding them along to ChatGPT, and after ChatGPT returns with a clearly defined goal, Auto-GPT turns back to ChatGPT, asking it to break this goal down into a a series of steps. Once ChatGPT has done that, Auto-GPT then asks ChatGPT to translate each of these steps into a sequence of actions.
These actions can either be executed by Auto-GPT directly – Auto-GPT will instruct ChatGPT to write code that fulfills those actions, if they can be performed entirely within the computer – or it can request that such-and-such an action be performed in the real world, with the results being fed back into Auto-GPT once that action has been completed. Auto-GPT will perform each of these actions in the correct order, and should the process fail at any point, will consult with ChatGPT about how it might perform that action differently, then try that new action, doing this again and again until it finds an action that succeeds. Auto-GPT does this until all the steps have been performed – and the task completed.
That a computer can perform this sort of planning around goal execution is not surprising; numerous AI problem-solving systems have existed for decades. What makes Auto-GPT remarkable is that it is both very capable – it rarely fails to complete the task – and entirely driven by human interactions in plain English. This means that pretty much anyone who can type can ask Auto-GPT to work for them as a problem solver. A perfect assistant.
It’s still very early days for these autonomous agents. They’re very powerful, yet also have one obvious shortcoming: they’re native to the purely digital world of computers and the Internet. Getting them to do something in the real world means we’ll need to connect them to all of the systems and sensors we’ve strung around our increasingly-intelligent planet. And not just connect them, but give them the permissions they need to control our world. That permission will come, as we find all the ways autonomous agents can help us shoulder the burden of managing an increasingly complex world.
This is where people start to imagine HAL-9000 refusing to open the pod bay doors, or – god forbid – Skynet launching the nukes. We needn’t worry about that. Although these systems are autonomous, they aren’t in any way conscious. They have no desires, and no volition – beyond working their hardest to perform whatever task you’ve set them to.
Autonomous agents are already very useful for tasks such as gathering data about the operations of a business or business unit, or sifting through a data ‘lake’ – highlighting the most interesting bits for review. And let’s not overlook the tremendous value of having an assistant keen and capable of helping us plow through the mountains of paperwork generated by every organisation.
Will autonomous agents be looking to take our jobs? No. But if we keep them busy, they’ll help us do better in ours.