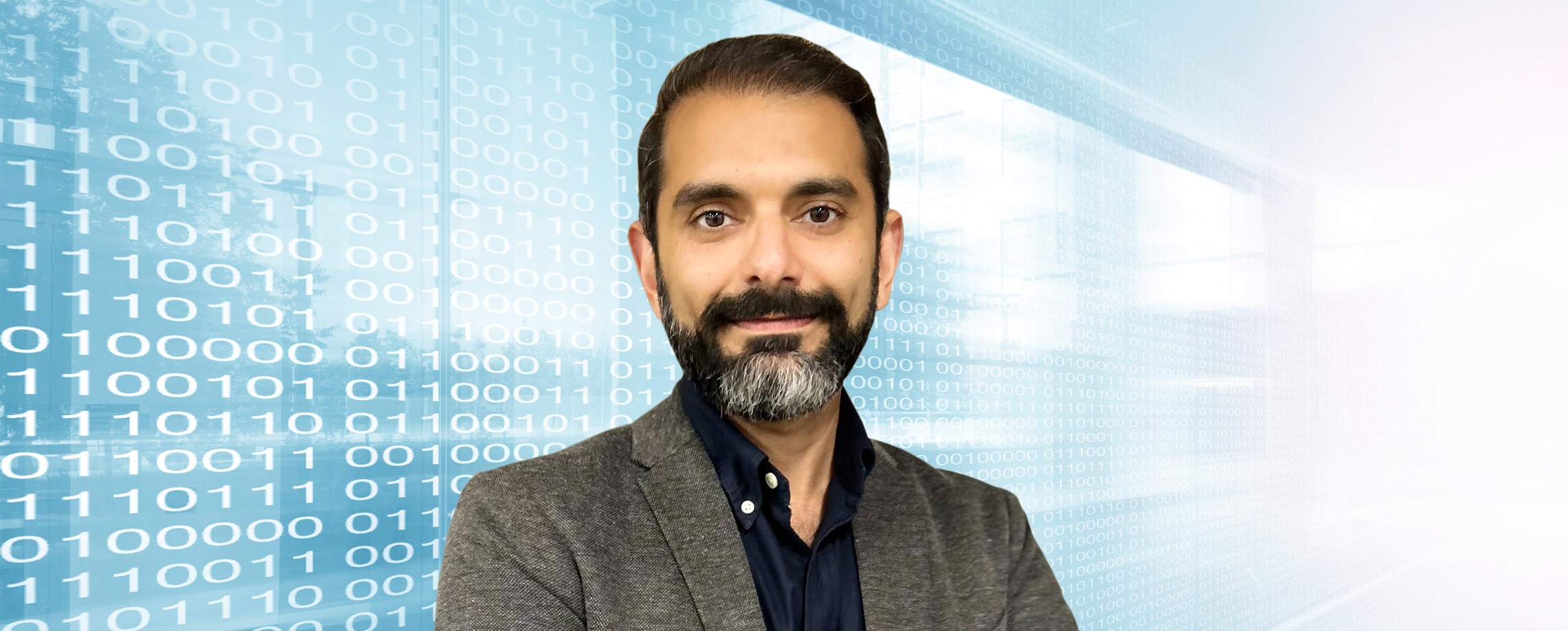
- Author: Tariq Munir
- Posted: August 13, 2024
Solving CFO’s Data Problem
Do not start a Data Lake project without these 3 things!
Unification, Harmonization, and Democratization of Data is the North Star for every CFO’s Digital Transformation agenda….and rightly so. It is considered the holy grail of building an Advanced Analytics capability. A global Data Analyst’s survey revealed that 98% of companies today are using Business Intelligence tools for decision-making. No one can argue the importance of a well-grounded, unified, and harmonized data source for their organization. Whether to use it for data visualization and reporting capability or building the Advanced Analytics capability. It is the natural right priority for any CFO.
Despite the right focus and many multi-year enterprise data unification projects, data quality in most organizations is still mediocre at best. As per a survey, 86%-90% of Data Analysts say that either they had to use data that was out of date or simply unreliable. Siloed business processes, below-par M&A integrations, and disparate technology architecture are among the many reasons to blame. Many Finance functions witness their pristine Data Lakes turning into more of data puddles or swamps.
To avoid such a dilemma, CFOs need to consider three critical aspects of any Data Lake project:
Start with Why >
Yes, the famous work by Simon Senik is also relevant to building a Data Lake. Before embarking on any Data Lake project or otherwise committing resources to it, ask the question, ‘Why do we need a Data Lake?’. It goes back to the premise of how any Digital initiative needs to be anchored into solving specific business problems, building value-based use cases, and how it will impact the way businesses can create and deliver value.
Starting with Why ensures that every decision you are taking either before or during a Data Lake project is tied to the business issue and not just a hype-cycle need. Multiple times a relatively simple data set in Power BI (for instance) is good enough for some of the business use cases.
Now, you will almost always be tempted to add all the data the organization generates or has access to within a Data lake. Beware of this temptation as it only complicates a seemingly simple initiative. To avoid this trap, create an inventory of existing Data sources and identify which ones will deliver the most value (understand the ‘Why’ of Data Lake). You might just be left with a few most relevant ones e.g. Data sources ERP (for actual financial data), Reporting systems (for mapping onto Statutory and management reporting), FP&A systems (for Forecast and budget data), CRMs and Secondary sales data. The Pareto Principle works perfectly here…focus on those 20% of the data sources that deliver 80% of targeted business value in terms of insights generation and use cases.
From there onwards, provided there is a strong architecture and governance framework, ingesting new data sources or previously unused ones would be easier, delivering exponential value.
Prioritize Knowledge retention >
One of the top reasons Data Lakes often turn into muddy puddles is a lack of knowledge transfer during process handovers, technical upgrades, and people changes. To address that a “Data Knowledge Catalogue” needs to be at the core of your Data Lake project.
A “Data Knowledge Catalogue” is similar to a library catalogue, offering detailed information about the data, such as its source, format, contents, owners, and how it can be accessed and used. This Catalogue enables data analysts and their teams to identify, extract and use the data. This step needs to start from the pre-implementation phase to pretty much indefinitely. These fields are generally referred to as “metadata” since they are not part of the data itself but are only used to categorize and label the data.
Some examples of metadata include Access Control lists (ACL), Source, Update timestamp, Origin, Data Dependencies, Usage statistics, Data Asset Tags, and so on. You do not need to know every field, however, work closely with the Dev team to ensure that an almost unproportionate amount of importance is given to this step.
It’s not about Data >
A relatively easier part of any Data Lake project is its technical architectures and data ingestions. It might seem contrarian at the beginning but when compared with the Organizational culture, Governance, and Privacy aspects…you will agree.
Like any digital transformation initiative, focusing on a data-driven culture, digital mindset, and Governance is critical. The underlying principle of a data lake is to enable the democratization of data and insights. This would effectively mean, most of the time, the information will be available to people in the organization at the same time as yourself. This might create a sense of lack of control over the data and insights. Since the flow of information is rapid and widely available, you might not know the answers to all the questions arising from it. A data-driven and digital mindset enables the organization to be ok with ambiguity. Leaders acknowledge that they don’t have all the answers and are open to exploring further insights based on data. Building this mindset is critical as otherwise, teams tend to fall back to old ways of working where they try to massage data to match the intuition or a preconceived narrative.
Additionally, establish a Balanced Scorecard to measure and track the progress of the Data lake project. Establish cross-functional Steering Committees responsible for the overall direction and completion of the project. SteerCo must provide support to the project team in ensuring any roadblocks are cleared and resourcing needs are addressed. Further, define and lead with clear Roles and responsibilities and a Reward & recognition system. A functional SteerCo does not just delegate the tasks that come up during the progress meetings, but actively solicits support from relevant stakeholders and owns the successes, failures, and opportunities arising.
Data unification is a highly complex and resource-intensive, yet essential initiative. It is a baseline to kick-start the ML/AI Deployment across the organization. With a careful understanding of the business value it generates (the ‘Why’), robust metadata, and most importantly, prioritizing the human side of this initiative, there’s no reason why one can’t succeed.