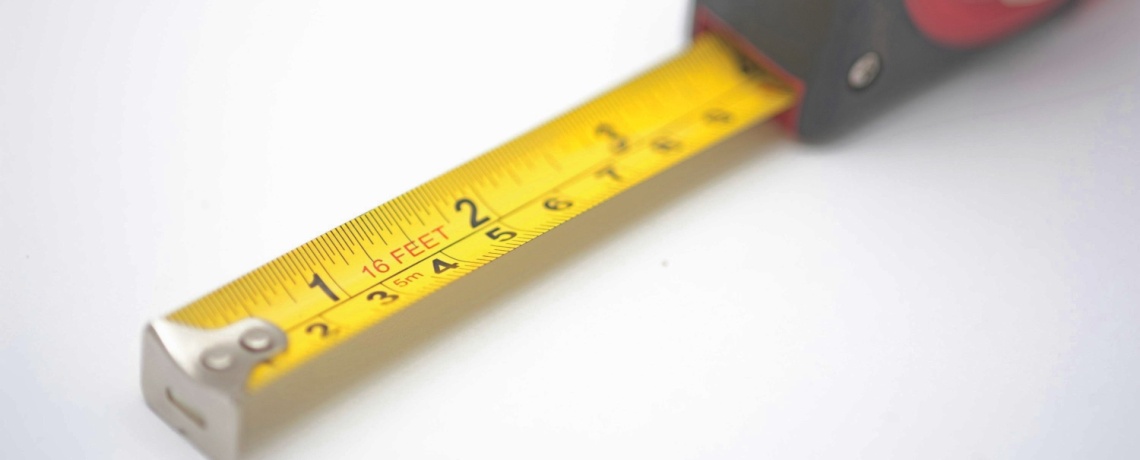
- Author: Tariq Munir
- Posted: October 8, 2024
Measuring the Elusive ‘Return on AI Investment’
How CFOs can avoid common pitfalls
A recent study by Gartner suggested that 80% of the CFOs will be increasing their spend on AI in the next two years.
There is no debate on the potential of such an investment to bring significant value from transforming business operations to redesigning customer experiences. However, when the question comes to quantify this ‘significant value’, business leaders struggle to find an answer. A PwC Pulse survey revealed as high as 88% of business leaders struggle to measure the return on their digital investment.
This is a huge problem. If we cannot measure the return, the ability to prioritize the investment behind the right AI project becomes a question mark. Similarly, monitoring and thus governing the investment becomes a challenge.
An AI deployment is not linear — unlike a conventional system implementation with clear go/no-go decision — AI continues to evolve over time. Once the first-cut model is put into use, it generates more data, more data means better algorithm (in most cases), better algorithm means more usage, and the cycle continues. With that the potential value it can generate, and the cost it incurs also increases.
To address this dilemma, CFOs and business leaders cannot just rely on conventional measures like NPV, IRRs, and Payback periods, nor doing it once at the start of the project approval time will suffice. This requires a balanced approach towards defining key metrices of success and monitoring those throughout the AI product Lifecyle.
Here are 3 ways CFOs and their Executive teams can measure, monitor, and track progress against their AI investments:
Define clear deployment goals:
AI investment starts with a clear deployment goal. This seems like an intuitive step but is often overstepped in the rush to kick-off the project. General statements like ‘improved forecast accuracy’ or ‘enabling data-driven decision-making’ are too vague. The AI model performance depends upon this goal as it determines our comfort-level with model’s accuracy right from the beginning. Important to understand here is that AI model’s accuracy is a trade-off between cost of training the model and the risk we are accepting for a wrong output.
CFOs need to collaborate extensively with their cross-functional teams to define what would success look like for the model to be deemed accurate along with the end-to-end impact it creates. For instance, a deployment goal to predict machine failure 80% of the times might mean 10% reduction in maintenance cost year on year. However, it is critical to factor in the cost of maintaining, updating and re-training the model as it gets trained on additional data generated from actual failures. Researchers at Stanford University found out that the cost of training AI models doubles every 9 months!
Create a Balanced Scorecard view:
AI model’s accuracy is just one of many measures to look at. In fact, it can be a misleading metric to track. A high accuracy does not mean better model, or as we noted above, the most cost-effective model. The conventional Financial measures like simple ROI (Net benefit/cost), NPV, and IRR over AI lifecycle are some of the KPIs you will typically need to have for both AI or non-AI projects. However, in addition to those it is vital to create a balanced view by adding Business Performance KPIs. These Business performance KPIs are sometimes not directly related to financial benefit but indirectly increases revenues, reduces costs, or avoids losses. For instance, speed-to-market of a new product, number of increased sales calls per sales rep, speed-to-insight, speed-to-analytics, and so on.
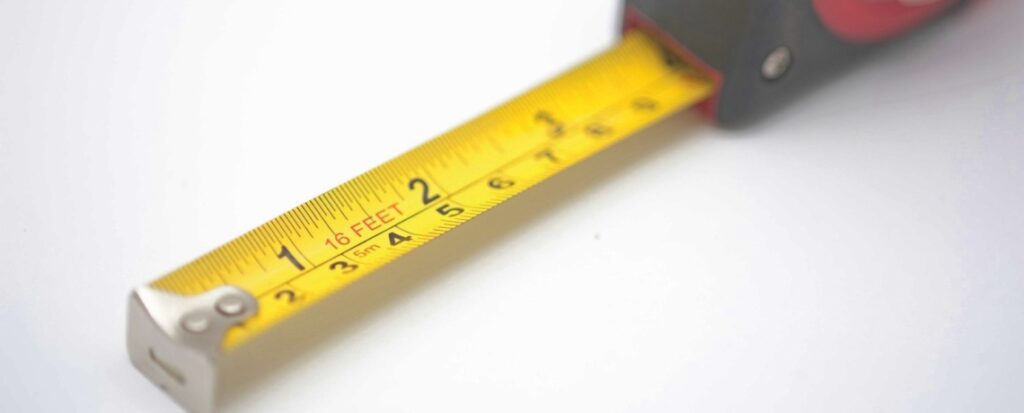
Now it does not end here. Imagine a situation where you have an 80% accurate model and an ROI of 20%, but the model is not user friendly and is making your team stay late to build work arounds. This will reflect directly on the team’s engagement scores. So would we still call this project a success and delivering the right return. Therefore, adding Non-Financial measures is equally important. Organizational engagement scores, Net promoter Scores, Adoption rates etc. are a few examples of such KPIs.
Continuous monitoring:
No one said AI implementation was going to be easy. Just having KPIs is not enough. A robust monitoring framework needs to be in place as well to ensure tracking of the KPIs we noted in point 2. This needs to happen throughout the AI development, implementation, and deployment stages. This will also make certain that a clear responsibility and accountability system is in place. A best practice is to have a Digital Streeting Council or Committee in place that meets regularly to review the portfolio of all Digital initiatives and their related returns. It is also important to monitor the health of overall portfolio of AI initiatives through these dashboards. This helps prioritize and direct resources and efforts to the correct AI projects with the right returns.
It is easy to lose momentum and enthusiasm in AI projects if leadership cannot measure and monitor the return on their investment. This leads to poor allocation of resources, a decline in Executive support, and eventually failed AI initiatives. By carefully defining their AI deployment goals, designing related KPIs, and establishing a robust monitoring framework around the AI investment can empower CFOs to avoid many of these pitfalls.