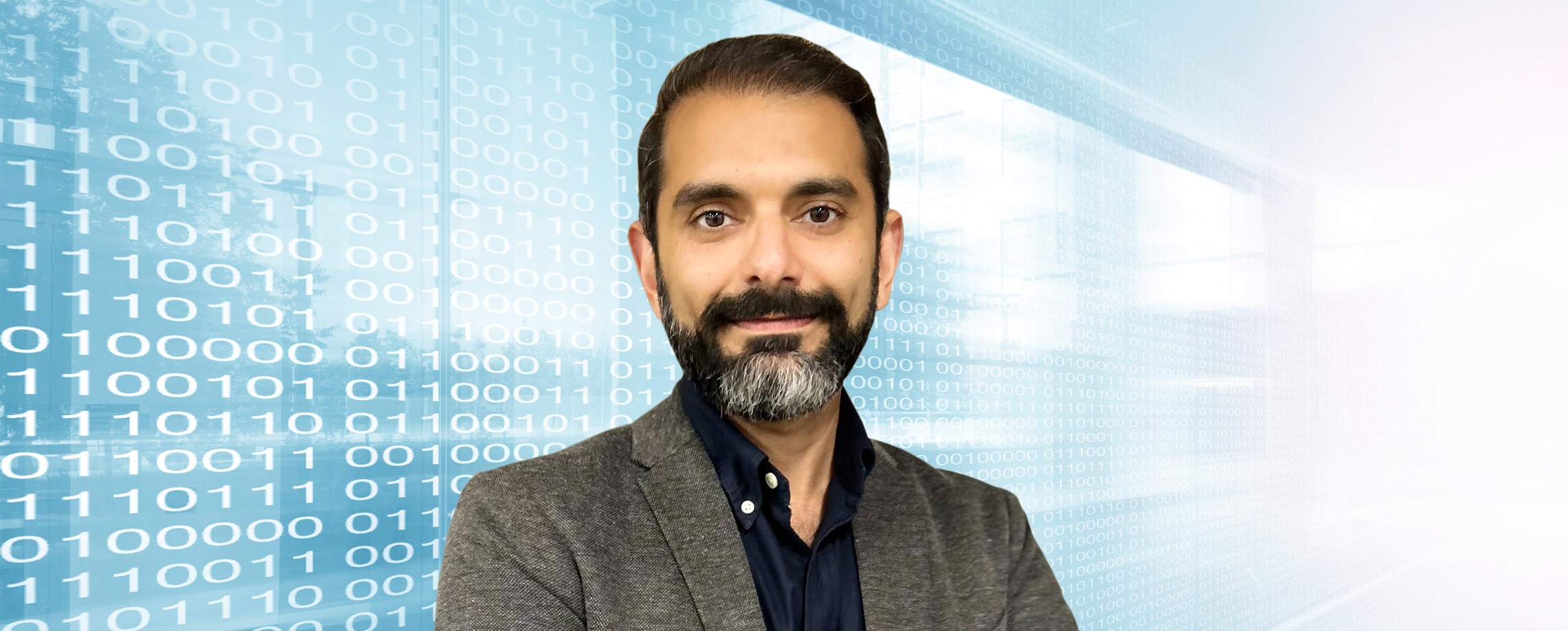
- Author: Tariq Munir
- Posted: November 19, 2024
CFO’s Digital Horizon: Five AI trends to watch in the next 5 Years!
An unprecedented amount of investment is going behind AI. International Data Corporation (IDC) predicts global spending on AI to reach $632 billion by 2028. The last couple of years have been a renaissance moment for AI mainly driven by cheap, virtually unlimited cloud computing and our ability to generate, store, and categorize scores of data.
Now before we explore how this wave of AI is likely to shape up Finance functions in next 5 years, let me clarify one thing. Neither this article is about predicting a dystopian future where humanoid robots are taking over the world nor am I a futurist. What I am sharing is, based on my research and experience, certain trends that I foresee will be re-architecting the core of how business and finance functions of the future operate.
Generative AI reality check >
As the hype settles in, business leaders are increasingly questioning the ROI and enterprise-specific use cases of Gen AI. In my last article, we discussed how measuring the return and controlling costs of AI is becoming more and more challenging. For the next few years, businesses will shift focus from the glitzy LLMs to less-sexy traditional Machine Learning. This does not mean a total pause on Gen AI, but we will move towards a convergence of AI technologies. As more processes gets automated using traditional machine learning, Gen AI will provide an intractability layer between CFOs and the core processes like Financial Planning, AP, AR, and so on.
Riches to RAGs >
There are several concerns around LLMs. Hallucinations, non-contextual responses, irrelevant output or simply incorrect results. Especially for Finance, where accuracy is the bread and butter, utility of generic Gen AI models is often met with scepticism. In next few years, as enterprise-level Gen AI evolves further, we will see a shift towards RAG or Retrieval-Augmented Generation. It is a technique of optimizing LLM outputs by using knowledge base outside of its training data sources before generating a response — thus grounding its output into factual, relevant and most-recent information. Think about an AI assistant having access to the data inside the organization within a protected environment while using the power of LLMs to generate accurate and context-aware responses. While we see a lot of experiments and pilots, some work still needs to happen in elevating current Finance AI maturity before these can be deployed in a scalable way.
Agentic AI >
The closest we can get to autonomous AI as of today is through a groundbreaking advancement called Agentic AI. It is an iterative reasoning AI technique that can make decisions and take actions with minimal human input. unlike Generative AI where it only responds to one prompt at a time, agentic AI possesses a degree of autonomy to make decisions, take actions, and learn to achieve the goal defined by human. It is suitable for non-rule based and complex tasks involving various interdependencies. For instance, when given an objective to perform month-end, it will not just post journals but will interact with various stakeholders to get their input, convert those into closing journals, perform reconciliations, and then monitor progress. Theoretically, it will involve minimal human supervision. This however, will be the next phase of automation as enterprise processes become mature and integrated.
Process Digital Twins >
A process digital twin is effectively a virtual replica of an entire process or system. Now I have written about Digital Twins in the past and it is not really a new thing as it has been there for a while when it comes to a virtual replica of physical products or plants. However, as process, data, and task mining becomes more sophisticated and intelligent, process Digital Twins will be the natural next phase of scenario planning, process intelligence, and streaming analytics.
As organisations becomes better at data governance and embedding advanced analytics, we will see an increasing number of discrete processes being virtualized. For instance, stage-gate innovation process and Integrated Business Process (IBP) might be the first few candidates.
Responsible & Ethical AI > As AI adoption matures and integrates into how organisations capture, create, and deliver value to its customers — the transparency, interpretability, privacy, avoiding bias, and ensuring compliance with new regulations will become an important part of CFOs role. Now I am not saying it will be the sole responsibility of CFO but, owing to her unique position at C-suite, she will play a major role in building a culture of proportionate ownership across the organization. Moreover, CFOs will be a key contributor and drivers in drafting the internal policies on responsible and ethical AI practices.
So, how can CFOs get ready?
The change is ubiquitous. The only option for us is to embrace and use it as an opportunity to unlock new customer experiences and redesigned operating models. While it would need a full discussion in itself, it is important to prioritize few actions for 2025, to be ready for next five years:
AI-readiness Assessment: Understand the current tech, data, processes, and people capabilities to evaluate the AI-readiness. Collaborate with reliable and experienced external partners to do that, leveraging their industry knowledge.
Identify business needs: This is the minimum you could do. Create a register of business needs, opportunities, and threats. Be tech-agnostic at this stage.
Map AI capabilities: Evaluate which AI capabilities are needed and the existing ones you already have in the broader organization, to address the business needs.
Prioritise quick wins: To create momentum, build organisational excitement, and solicit wider support, build and prioritize quick wins. Start a couple of high-impact, low-effort projects which can be completed under within 3-6 months.
Iterate: Be agile and strive for excellence as opposed to perfection. Go for Minimum Viable Products (MVPs) as opposed to trying to cover too many bases. Launch, learn, and iterate.
Build a holistic plan for AI literacy: Underpin all your efforts with a holistic upskilling, re-skilling, and de-skilling plan for Finance teams. Lead from the front and build a culture of continuous learning and taking initiatives based on that. Network and engage with like-minded professionals to lean about how other companies are approaching AI.
There is no deterministic view of future that can be presented here. Especially when so many powerful general-purpose technologies are changing it all the time. The only thing we should be focused on is our willingness to embrace the change, have a growth mindset, and think critically as to how technology can help solve some of the most pressing business problems and while doing so, create new avenues of growth.
About the Author – Tariq Munir
Tariq Munir is a thought leader on AI, Data, and its intersection with business. He is also a keynote speaker, trainer, and monthly columnist at CFO Magazine ANZ.
He can be reached at hello@tariqmunir.me or www.tariqmunir.me