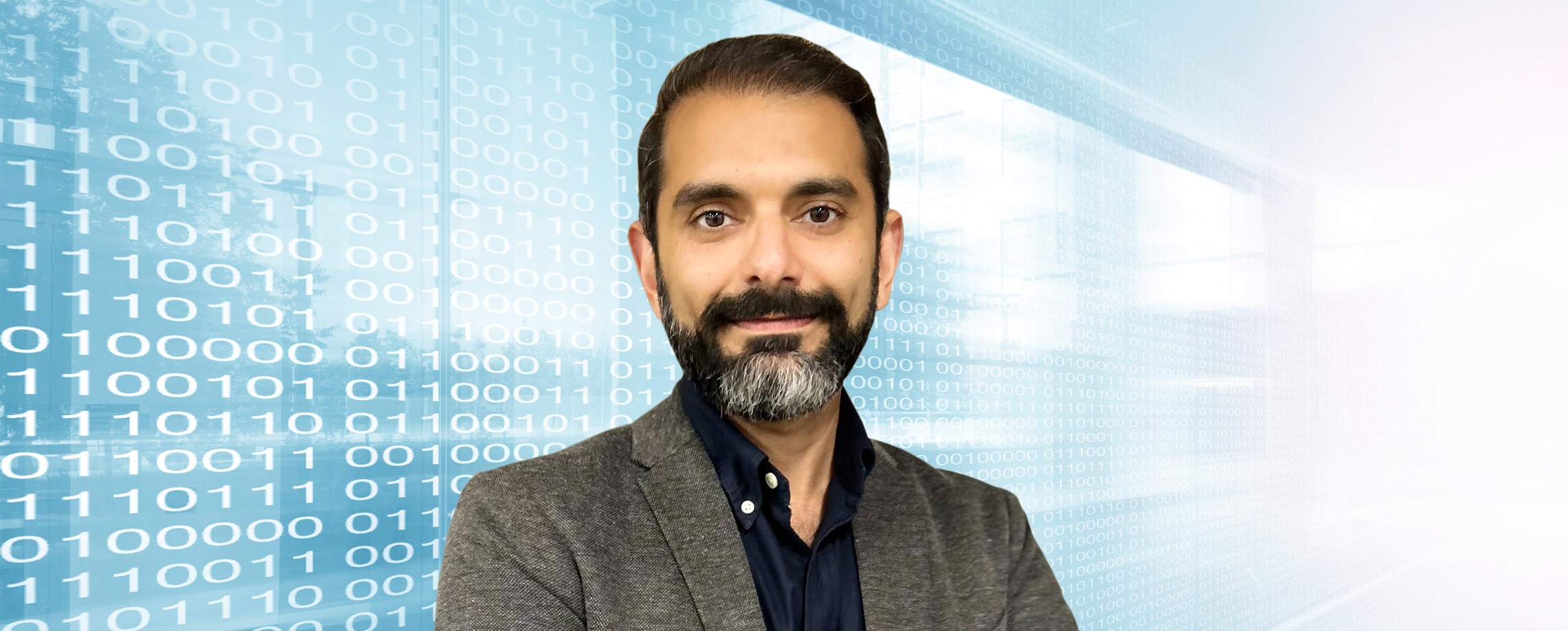
- Author: Tariq Munir
- Posted: January 22, 2024
4 Reasons Why Every CFO Needs to Have a Data Strategy in 2024
We are all excited about the dizzying pace of AI evolution and its prospects in business. Countless articles, podcasts, and videos are generated every minute promising the utopian ‘Data-driven’ decision making future. Organisations tend to go through a typical hype-cycle where initial possibilities excite every Executive till it reaches the peak. At that point, the implementation and adoption bottlenecks and the excitement gets replaced with frustration and internal conflicts.
Enabling Data-driven decision-making at scale is more than just implementation of cutting-edge technology. It is about creating a Data-driven culture. A 2023 MIT Sloan Management Review Survey revealed that only 21% of surveyed data executives were able to develop a Data Culture within their organization. Now to build such a culture, an overarching Data strategy grounded in how to capture, classify, transform, and cleanse data is needed.
In fact, OpenAI’s COO in an interview last month, treated the common notion of AI bringing substantive business change as an ‘over-hype’ and admitted that the technology is still in its infancy. However, this brings a huge opportunity for organisations, especially their CFOs, to play a much bigger role in devising and influencing organisational Data strategy and be AI-ready in 2024. It kind of gives us a grace period before the real AI impact is felt across enterprise.
Due to its increasingly decentralised influence and involvement in commercial decisions, Finance leaders are best placed to focus their efforts on building a robust Data strategy in 2024. This would mean CFOs collaborating and co-creating with other C-suite to establish frameworks and processes around capturing, transforming, and governance of data.
Here are four such considerations every CFO must have in their Data Strategy in 2024:
Understand the Data landscape and business objectives
The first step is to take stock of your existing data sources across the organization and the key goals and objectives you are trying to achieve. Anchor the Finance Data strategy to a wider Business strategy and vision. Collaborate with CDOs or CIOs and expand beyond just financial data to incorporate non-financial information which eventually will enable an integrated financial forecasting and scenario planning capability.
The future of data-driven decision-making is building a Scenario Planning capability, which needs data more than just finance. An European Software company used 25+ data sources — ranging from Pricing to Climate Change, Taxes, and Regulatory data — to create a strong Scenario planning capability.
Data Capture and Transformation
Not all Data is created equal. Depending upon the business case needs and size of the organization, there should be clear models and processes in place to capture, harmonize, and then consume the data. A best-in-class approach is to establish a Data Lake which captures both structured and unstructured data. The flexibility and scalability of such a data lake enable future deployment of AI/ML and other advanced analytics capabilities.
However, as for any digital initiative, anchor all data harmonization initiatives to a specific business need. Do not kick off an organisation-wide data unification project right from the beginning. It might seem tempting to keep adding data sources to a Data Lake project…as eventually every data is important in one way or the other. However, clearly understand the data relevant to the business case and only focus on that in the early phases. Start small and go incremental.
Ensure best practices are followed in any such project which includes things like establishing a Data Knowledge Catalog (a directory of data sources, its ownership, origin, etc.), a modular data architecture enabling flexibility and scalability, and a robust Governance framework.
Single Source vs Multiple Versions of Truth
The primary goal of any Data Strategy is to have a Single Source of Truth (SSoT). It is equally important that the same SSoT enables Multiple Versions of Truth (MVoT). SSoT works at Data while MVoT transforms the same data into actionable insights in a given business context. For example, an SSoT ensures that Customer Master Data is harmonized (e.g. eliminating duplicate Customers, and correct grouping by Channel) which in turn allows your Accounts Receivable function to correctly match Invoices with Promo claims. On the other hand, an alternate version of the same data would enable Key Account Managers to analyze Promo performance by customer i.e. creating an MVoT using the same SSoT.
Striking a balance between SSoT and MVoT should be a key consideration, which will depend on several factors like use cases, granularity, control, and its ability to drive data-driven decision-making.
Design for Privacy and Security
Make Security and Privacy a foundational part of any Data discussion right from the beginning. Depending upon where the data is stored — on-premise, public or private cloud, or a hybrid one — your data security needs and strategies will tailor accordingly. Similarly, how regulated the industry you operate in will be a key input to the Data strategy.
The estimated cost of one data breach is $4.3 million. Loss of goodwill and reputation is on top of that. Therefore, keep data security as paramount. Democratization of data does not mean everyone is given access to everything. Ensure a robust Data governance framework is in place which restricts the access privileges. Any sensitive, regulatory, or private data needs to be stored in an even more secure zone.
We started our discussion about having a strong Data Strategy to be able to be AI-ready in 2024. However, be aware of the privacy laws around the use of data for Algorithm training purposes. Just because an organization has the data, does not mean they can use it to train AI models. An increasing number of regulations prevent organisations from doing so without obtaining a specific consent. In January 2021, the Federal Trade Commission (FTC) in the US imposed a penalty known as “algorithmic disgorgement”. A notable case involved Everalbum Inc. and its photo storage ‘Everapp’. Customers’ photos and data stored in the app were being used to train its facial recognition algorithm…without their consent. The algorithm was later on marketed to its enterprise customers. FTC directed the company to destroy a huge amount of data including photos, algorithms, and models due to these privacy violations.
No one can predict with certainty how AI will impact business and work — especially when the present is so disruptive and volatile. However, one thing is certain…what we do today defines what the future is going to look like.
Embedding a holistic Data Strategy within the Organisation is that right step CFOs can influence and drive today to ensure the future (especially for Finance) is defined by a truly Data-driven decision-making culture.
This is how we can empower Finance to play a much bigger role in 2024 and beyond — i.e. be the Growth Drivers and true Strategic Business Partners to the entire organisation.